Om Thakkar
Member of Technical StaffOpenAI
San Francisco, CA, USA
Email : om "at" openai.com
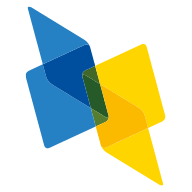
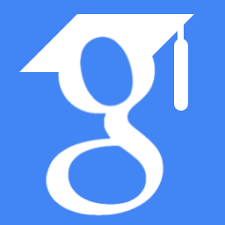
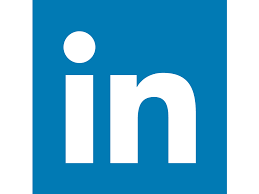
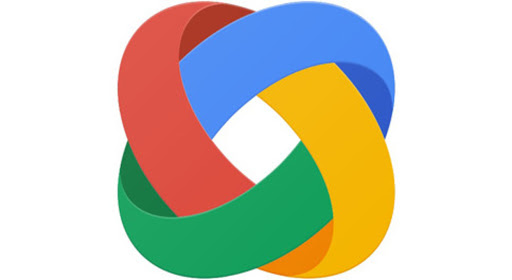
Short Bio
I am a research engineer at OpenAI, working on privacy research in the team of Vinnie Monaco. My research is in privacy-preserving AI, with a specific focus on differential privacy and its applications to deep learning in production systems (including language and speech models).Before joining OpenAI, I was a senior research scientist at Google, where I worked for 5 years. Prior to Google, I graduated with a Ph.D. in Computer Science from Boston University (BU) in 2019. I was very fortunate to be advised by Dr. Adam Smith. At BU, I was a part of the Security group, and the Theoretical Computer Science group. I completed the first 3.5 years of my Ph.D. in the Department of Computer Science and Engineering at
News
- Our paper titled Recycling Scraps: Improving Private Learning by Leveraging Intermediate Checkpoints has been accepted to appear at PETS 2025.
- Two papers (Efficiently Train ASR Models that Memorize Less and Perform Better with Per-core Clipping, and Quantifying Unintended Memorization in BEST-RQ ASR Encoders) (oral presentation) accepted to appear in Interspeech 2024.
- Two papers (Unintended Memorization in Large ASR Models, and How to Mitigate It, and Noise Masking Attacks and Defenses for Pretrained Speech Models) accepted to appear in ICASSP 2024.
- Our paper titled Why Is Public Pretraining Necessary for Private Model Training? has been accepted to appear at ICML 2023.
- Our paper titled Measuring Forgetting of Memorized Training Examples has been accepted to appear at ICLR 2023 .
- Our paper titled Recycling Scraps: Improving Private Learning by Leveraging Intermediate Checkpoints has been accepted to appear at PPAI 2023 (AAAI 2023) as an oral presentation.
Resume
- My most recent resume (last updated in March, 2025) can be found here.
Manuscripts
Patents
- Leveraging Intermediate Checkpoints To Improve The Performance of Trained Differentially Private Models. Om Thakkar, Arun Ganesh, Virat Shejwalkar, Abhradeep Thakurta, and Rajiv Mathews. Filed US Patent 63/376,528.
- Detecting Unintended Memorization in Language-Model-Fused ASR Systems. W. Ronny Huang, Steve Chien, Om Thakkar, and Rajiv Mathews. Published US Patent 2023/0335126.
- Generating and/or Utilizing Unintentional Memorization Measure(s) for Automatic Speech Recognition Model(s). Om Thakkar, Hakim Sidahmed, W. Ronny Huang, Rajiv Mathews, Françoise Beaufays, and Florian Tramèr. Published US Patent 2023/0317082.
- Server Efficient Ehnancement of Privacy in Federated Learning. Om Thakkar, Peter Kairouz, Brendan McMahan, Borja Balle, and Abhradeep Thakurta. Published US Patent 2023/0223028.
- Phrase Extraction for ASR Models. Ehsan Amid, Om Thakkar, Rajiv Mathews and Françoise Beaufays. Published US Patent 2023/0178094.
- Leveraging Public Data in Training Neural Networks with Private Mirror Descent. Ehsan Amid, Arun Ganesh, Rajiv Mathews, Swaroop Ramaswamy, Shuang Song, Thomas Steinke, Vinith Suriyakumar, Om Thakkar, and Abhradeep Thakurta. Published US Patent 2023/0103911.
- Ascertaining And/or Mitigating Extent of Effective Reconstruction, of Predictions, From Model Updates Transmitted in Federated Learning. Om Thakkar, Trung Dang, Swaroop Ramaswamy, Rajiv Mathews, and Françoise Beaufays. Published US Patent 2022/0383204.
- Mixed Client-Server Federated Learning. Françoise Beaufays, Swaroop Ramaswamy, Rajiv Mathews, Om Thakkar, and Andrew Hard. Published US Patent 2022/0293093.
Publications
Papers available here may be subject to copyright, and are intended for personal, non-commercial use only. Unless specifically indicated, all publications have authors listed in the alphabetical order of last names (as per the convention in theoretical computer science).- Recycling Scraps: Improving Private Learning by Leveraging Intermediate Checkpoints.
Virat Shejwalkar, Arun Ganesh*, Rajiv Mathews*, Yarong Mu*, Shuang Song*, Om Thakkar*, Abhradeep Thakurta*, and Xinyi Zheng*. (*Alphabetical order.) In Privacy Enhancing Technologies Symposium (PETS) 2025.
- Efficiently Train ASR Models that Memorize Less and Perform Better with Per-core Clipping.
Lun Wang, Om Thakkar, Zhong Meng, Nicole Rafidi, Rohit Prabhavalkar, and Arun Narayanan. (In order of contribution). In Interspeech 2024.
- Quantifying Unintended Memorization in BEST-RQ ASR Encoders. Virat Shejwalkar, Om Thakkar, and Arun Narayanan. (In order of contribution). In Interspeech 2024. Accepted for an oral presentation.
- Unintended Memorization in Large ASR Models, and How to Mitigate It.
Lun Wang, Om Thakkar, and Rajiv Mathews. (In order of contribution). In the 2024 IEEE International Conference on Acoustics, Speech, and Signal Processing (ICASSP 2024).
- Noise Masking Attacks and Defenses for Pretrained Speech Models.
Matthew Jagielski, Om Thakkar, and Lun Wang. (In order of contribution). In the 2024 IEEE International Conference on Acoustics, Speech, and Signal Processing (ICASSP 2024).
- Why Is Public Pretraining Necessary for Private Model Training?
Arun Ganesh, Mahdi Haghifam, Milad Nasr, Sewoong Oh, Thomas Steinke, Om Thakkar, Abhradeep Thakurta, and Lun Wang. In the Fortieth International Conference on Machine Learning (ICML 2023).
- Measuring Forgetting of Memorized Training Examples.
Matthew Jagielski, Om Thakkar, Florian Tramèr, Daphne Ippolito, Katherine Lee, Nicholas Carlini, Eric Wallace, Shuang Song, Abhradeep Thakurta, Nicolas Papernot, and Chiyuan Zhang. (In order of contribution) In the Eleventh International Conference on Learning Representations (ICLR 2023).
- Extracting Targeted Training Data from ASR Models, and How to Mitigate It.
Ehsan Amid*, Om Thakkar*, Arun Narayanan, Rajiv Mathews, and Françoise Beaufays. (In order of contribution) In Interspeech 2022. Accepted for an oral presentation. *Equal contribution.
- Detecting Unintended Memorization in Language-Model-Fused ASR.
W. Ronny Huang, Steve Chien, Om Thakkar, and Rajiv Mathews. (In order of contribution) In Interspeech 2022. Accepted for an oral presentation.
- Public Data-Assisted Mirror Descent for Private Model Training.
Ehsan Amid, Arun Ganesh, Rajiv Mathews, Swaroop Ramaswamy, Shuang Song, Thomas Steinke, Vinith Suriyakumar, Om Thakkar, and Abhradeep Thakurta. In the Thirty-ninth International Conference on Machine Learning (ICML 2022).
- A Method to Reveal Speaker Identity in Distributed ASR Training, and How to Counter It.
Abstract▼ Trung Dang, Om Thakkar, Swaroop Ramaswamy, Rajiv Mathews, Peter Chin, and Françoise Beaufays. (In order of contribution) In the Forty-Seventh International Conference on Acoustics, Speech, & Signal Processing (ICASSP 2022).
- The Role of Adaptive Optimizers for Honest Private Hyperparameter Selection.
Abstract▼ Shubhankar Mohapatra, Sajin Sasy, Gautam Kamath*, Xi He*, and Om Thakkar*. (*Alphabetical order.) In the Thirty-Sixth AAAI Conference on Artificial Intelligence (AAAI 2022). Accepted for an oral presentation.
- Differentially Private Learning with Adaptive Clipping.
Abstract▼ Galen Andrew, Om Thakkar, Swaroop Ramaswamy, and Brendan McMahan. (In order of contribution) In the Thirty-fifth Conference on Neural Information Processing Systems (NeurIPS 2021).
- Revealing and Protecting Labels in Distributed Training.
Abstract▼ Trung Dang, Om Thakkar, Swaroop Ramaswamy, Rajiv Mathews, Peter Chin, and Françoise Beaufays. (In order of contribution) In the Thirty-fifth Conference on Neural Information Processing Systems (NeurIPS 2021).
- Practical and Private (Deep) Learning without Sampling or Shuffling.
Abstract▼ Peter Kairouz, Brendan McMahan, Shuang Song, Om Thakkar, Abhradeep Thakurta, and Zheng Xu. In the 38th International Conference on Machine Learning (ICML 2021).
- Evading the Curse of Dimensionality in Unconstrained Private GLMs.
Abstract▼ Shuang Song, Thomas Steinke, Om Thakkar, and Abhradeep Thakurta. In the 24th International Conference on Artificial Intelligence and Statistics (AISTATS 2021).
- Privacy Amplification via Random Check-Ins.
Abstract▼ Borja Balle, Peter Kairouz, Brendan McMahan, Om Thakkar, and Abhradeep Thakurta. In the 34th Conference on Neural Information Processing Systems (NeurIPS 2020).
- Guaranteed Validity for Empirical Approaches to Adaptive Data Analysis.
Abstract▼ Ryan Rogers, Aaron Roth, Adam Smith, Nathan Srebro, Om Thakkar, and Blake Woodworth. In the 23rd International Conference on Artificial Intelligence and Statistics (AISTATS 2020).
- Advances in Privacy-Preserving Machine Learning.
Ph.D. Thesis, BU, September 2019. Supervisor: Dr. Adam Smith.
- Towards Practical Differentially Private Convex Optimization.
Abstract▼ Roger Iyengar, Joseph P. Near, Dawn Song, Om Thakkar, Abhradeep Thakurta, and Lun Wang. In the 40th IEEE Symposium on Security and Privacy (S&P 2019).
- Model-Agnostic Private Learning.
Abstract▼ Raef Bassily, Om Thakkar, and Abhradeep Thakurta. In the 32nd Conference on Neural Information Processing Systems (NeurIPS 2018). Accepted for an oral presentation.
- Differentially Private Matrix Completion Revisited.
Abstract▼ Prateek Jain, Om Thakkar, and Abhradeep Thakurta. In the 35th International Conference on Machine Learning (ICML 2018). Presented as a long talk.
- Max-Information, Differential Privacy, and Post-Selection Hypothesis Testing.
Abstract▼ Ryan Rogers, Aaron Roth, Adam Smith, and Om Thakkar. In the 57th Annual IEEE Symposium on Foundations of Computer Science (FOCS 2016).
Workshop Publications
- Training Large ASR Encoders with Differential Privacy.
Geeticka Chauhan, Steve Chien, Om Thakkar, Abhradeep Thakurta, and Arun Narayanan. (In order of contribution) In the 2024 IEEE Spoken Language Technology Workshop (SLT 2024).
- Differentially Private Parameter-Efficient Fine-tuning for Large ASR Models. Hongbin Liu, Lun Wang, Om Thakkar, Abhradeep Thakurta, and Arun Narayanan. (In order of contribution) In the 7th Deep Learning Security and Privacy Workshop (DLSP 2024), and the Theory and Practice of Differential Privacy Workshop (TPDP 2024).
- Recycling Scraps: Improving Private Learning by Leveraging Intermediate Checkpoints.
Virat Shejwalkar, Arun Ganesh*, Rajiv Mathews*, Om Thakkar*, and Abhradeep Thakurta*. In the Fourth AAAI Workshop on Privacy-Preserving Artificial Intelligence (PPAI 2023). Accepted for an oral presentation. *Alphabetical order.
- Public Data-Assisted Mirror Descent for Private Model Training.
Ehsan Amid, Arun Ganesh, Rajiv Mathews, Swaroop Ramaswamy, Shuang Song, Thomas Steinke, Vinith Suriyakumar, Om Thakkar, and Abhradeep Thakurta. In the Theory and Practice of Differential Privacy (TPDP) 2022 workshop (ICML 2022).
- Practical and Private (Deep) Learning without Sampling or Shuffling.
Peter Kairouz, Brendan McMahan,Shuang Song, Om Thakkar, Abhradeep Thakurta, and Zheng Xu. In the Theory and Practice of Differential Privacy (TPDP) 2021 workshop (ICML 2021).
- The Role of Adaptive Optimizers for Honest Private Hyperparameter Selection.
Shubhankar Mohapatra, Sajin Sasy,Gautam Kamath*, Xi He*, and Om Thakkar*. In the Theory and Practice of Differential Privacy (TPDP) 2021 workshop (ICML 2021). *Alphabetical order.
- Training Production Language Models without Memorizing User Data.
Swaroop Ramaswamy*, Om Thakkar*, Rajiv Mathews, Galen Andrew, Brendan McMahan, and Françoise Beaufays. (In order of contribution) In the Privacy Preserving Machine Learning (PPML) 2020 workshop (NeurIPS 2020). Accepted for an oral presentation. *Equal contribution.
- Privacy Amplification via Random Check-Ins.
Borja Balle, Peter Kairouz, Brendan McMahan, Om Thakkar, and Abhradeep Thakurta. In the Theory and Practice of Differential Privacy (TPDP) 2020 workshop (CCS 2020).
- Understanding Unintended Memorization in Federated Learning.
Abstract▼ Om Thakkar, Swaroop Ramaswamy, Rajiv Mathews, and Françoise Beaufays. (In order of contribution) In the PrivateNLP workshop (NAACL’21), the Theory and Practice of Differential Privacy (TPDP) 2020 workshop (CCS 2020), and the Privacy Preserving Machine Learning (PPML) 2020 workshop (NeurIPS 2020).
- Characterizing Private Clipped Gradient Descent on Convex Generalized Linear Problems.
Shuang Song, Om Thakkar, and Abhradeep Thakurta. In the Theory and Practice of Differential Privacy (TPDP) 2020 workshop, and the Privacy Preserving Machine Learning (PPML) 2020 workshop (NeurIPS 2020). Accepted for an oral presentation at TPDP 2020 (CCS 2020).
Interns
- Geeticka Chauhan (Summer'23-Fall'23, co-hosted with Abhradeep Thakurta)
- Hongbin Liu (Summer'23-Fall'23, co-hosted with Lun Wang)
- Virat Shejwalkar (Summer'22-Fall'22, co-hosted with Abhradeep Thakurta)
- Vinith Suriyakumar (Summer'21, co-hosted with Swaroop Ramaswamy)
- Trung Dang (Summer'20-Spring'21, co-hosted with Swaroop Ramaswamy)
Internships and Research Visits
- Visiting Graduate Student in the Data Privacy program at the Simons Institute, Berkeley during Spring'19.
- Research Intern at Google Brain, Mountain View, CA during Summer 2018. Mentors: Úlfar Erlingsson, and Kunal Talwar.
- Visiting Student Researcher at University of California, Berkeley, CA during Fall 2017. Host: Dr. Dawn Song.
- Research Intern at Google, Seattle, WA during Summer 2017. Mentors: Brendan McMahan, and Martin Pelikan.
- Research Intern in the CoreOS: Machine Learning team at Apple, Cupertino, CA during Summer 2016.
Talks and Poster Presentations
- Privacy Leakage in Speech Models: Attacks and Mitigations @ the UMass AI&Sec Security and Privacy seminar on March 31, 2025. (Slides)
- Training in Prod Federated Learning with Formal Differential Privacy
- @ the Privacy Paradox: AI and Digital Platforms elective, IIM-Ahmedabad, India on October 11, 2022. (Slides)
- @ the Privacy Paradox: AI and Digital Platforms elective, IIM-Ahmedabad, India on July 1, 2022. (Slides)
- @ the 2022 HAI Spring Conference on Key Advances in Artificial Intelligence on April 12, 2022. (Talk video)
- Part of the panel discussion on Accountable AI.
- Google AI blog post on which the talks were based.
- DP-FTRL in Practice @ the Data Privacy: Foundations and Applications Reunion workshop, Simons Institute, Berkeley on March 14, 2022.
- Practical and Private (Deep) Learning without Sampling or Shuffling @ the Boston-area Data Privacy Seminar on February 28, 2022.
- Understanding Unintended Memorization in Language Models Under Federated Learning
- @ the PrivateNLP 2021 Workshop (NAACL 2021) on June 11, 2021.
- @ PPML2020 Workshop (NeurIPS 2020) on December 11, 2020.
- @ TPDP 2020 Workshop (CCS 2020) on November 13, 2020.
- Towards Training Provably Private Models via Federated Learning in Practice
- @ PPML2020 Workshop (NeurIPS 2020) on December 11, 2020.
- @ the Workshop on Federated Learning and Analytics 2020 (Google) on July 29, 2020.
- Characterizing Private Clipped Gradient Descent on Convex Generalized Linear Problems
- @ PPML2020 Workshop (NeurIPS 2020) on December 11, 2020.
- @ TPDP 2020 Workshop (CCS 2020) on November 13, 2020.
- Privacy Amplification via Random Check-Ins
- @ NeurIPS 2020 on December 8, 2020.
- @ TPDP 2020 Workshop (CCS 2020) on November 13, 2020.
- @ the Ph.D. Intern Research Conference 2020 (Google) on July 22, 2020.
- Towards Practical Differentially Private Convex Optimization
- @ the Future of Privacy Forum booth, Global Privacy Summit 2019, Washington, DC on May 3, 2019. (Coverage)
- @ the Privacy Tools Project meeting, Harvard on March 5, 2018.
- Model-Agnostic Private Learning,
- @ the 2019 IEEE North American School of Information Theory (NASIT), held at BU, on July 3, 2019. (Poster)
- @ the 2018 Open AIR: Industry Open House, BU on October 12, 2018. (Poster)
- Building Tools for Controlling Overfitting in Adaptive Data Analysis, @ the Adaptive Data Analysis workshop, Simons Institute, Berkeley on July 7, 2018.
- Differentially Private Matrix Completion Revisited
- @ the Mathematical Foundations of Data Privacy workshop, BIRS on May 2, 2018. (Talk video)
- @ the BU Data Science (BUDS) Day, Boston University on January 26, 2018. (Poster)
- @ the Privacy Tools Data Sharing workshop, Harvard University on December 12, 2017. (Poster)
- @ the Security Seminar, UC Berkeley on October 9, 2017.
- A brief introduction to Concentrated Differential Privacy, @ CSE Theory Seminar, Penn State on April 14, 2017.
- Max-Information, Differential Privacy, and Post-selection Hypothesis Testing
- @ INSR Industry Day, Penn State on April 24, 2017. (Poster)
- @ SMAC Talks, Penn State on December 2, 2016.
- @ CSE Theory Seminar, UCSD on November 7, 2016.
- @ CSE Theory Seminar, Penn State on October 14, 2016.
- Max-Information and Differential Privacy, @ CSE Theory Seminar, Penn State on May 5, 2016.
- The Stable Roommates Problem with Random Preferences, @ CSE Theory Seminar, Penn State on April 10, 2015.
- The Multiplicative Weights Update Method and an Application to Solving Zero-Sum Games Approximately, @ CSE Theory Seminar, Penn State on November 3, 2014.
Teaching
- Teaching assistant:
- CMPSC 465 Data Structures and Algorithms, Spring 2017 @ Penn State.
- CMPSC 360 Discrete Mathematics for Computer Science, Spring 2015 @ Penn State.
- IT 114 Object Oriented Programming, Spring 2014 @ DA-IICT.
- IT 105 Introduction to Programming, Fall 2013 @ DA-IICT.
Professional Activities
- Program committee member for AAAI 2025, TPDP (2020, 2022, 2024-2025).
- Reviewer for journals: Information Sciences 2024, T-IFS (2019, 2021-2022), JPC (2019, 2022), JSSAM 2021, TSC 2020, JMLR 2018.
- Reviewer for conferences: ICML (2018, 2021-2024), ASRU 2023, NeurIPS (2019-2023), RANDOM 2023, AISTATS 2022, S&P (2017, 2019, 2022), PETS (2017-2021), IJCAI 2019, CCS (2018-2019), STOC (2016, 2018), ACSAC 2017, FOCS 2017, WABI 2015.
- Reviewer for NIST's The Unlinkable Data Challenge: Advancing Methods in Differential Privacy.
Recent Awards
- Received a travel award for S&P 2019.
- Received a travel award for NeurIPS 2018.
- Received a travel award for ICML 2018.
- Received a GSO Conference Travel Grant for Summer 2018.
- Received a registration award for FOCS 2014.
Miscellaneous
- Report on Node-differentially Private Algorithms for Graph Statistics. It includes joint work with Ramesh Krishnan.